Impact on a Large Scale
The Importance of Evidence
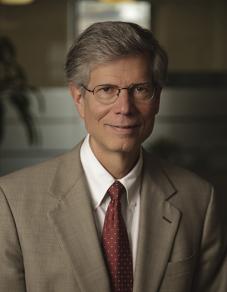
Adapted from remarks made to the Growth Philanthropy Network/Social Impact Exchange 2014 Conference on Scaling Impact.
The conference’s theme describes the key challenge: if philanthropic organizations are going to make a difference, they will need to do so for large numbers of people:
- 46 million Americans live in poverty, of whom 16 million are children.
- 10 million Americans are unemployed, of whom 3.4 million are counted among the long-term unemployed.
- 2.4 million Americans are incarcerated, many of them young men.
Making a meaningful difference means not just ameliorating these problems but solving them, not just treating symptoms but addressing underlying causes.
To get there, the conference sessions highlight three promising strategies that are generating a lot of buzz in 2014:
- Collective Impact: The idea that if many organizations all pull in the same direction, doing a better job of networking, coordinating, and sharing the insights they gain and the lessons they learn, their collective effect will be greater than the sum of their individual contributions.
- Social Impact Bonds (SIBs): The newest form of impact investing, in which nonprofit service providers get much-needed capital both to innovate and to expand. Governments only pay if programs achieve agreed-upon performance goals, and investors receive both an investment return and a social return.
- Social Movement Building: Comprehensive strategies to link policy, programs, advocacy, and on-the-ground action, mobilizing dispossessed groups to motivate the integration of policy, practice, and politics.
For any of these strategies to make a difference on a large scale, however, the underlying programs — what participants actually get — must be effective. There can be no collective impact if the individual programs being collected are not effective. The additive effect of multiple programs with no impact is still 0. As the intermediary for the first SIB in the United States — New York City’s program for ex-offenders on Rikers Island — and the original evaluator of the ex-offender reentry program at the center of New York State’s first SIB, MDRC has a deep interest in this new investment vehicle. But if one focuses only on the potential for all players to win in a successful Social Impact Bond, one can miss the reality that an unsuccessful program funded with a Social Impact Bond has no winners: not the government entity involved, not the participants, not the investors, and not the nonprofit organization operating the program. Moreover, if effects are not measured reliably, the government entity can end up paying for ineffective services that do not save money and that benefit neither clients nor society at large. Finally, social movements can change laws and open up opportunities, but to change lives they will still need to build on strategies that actually work, which means that they must privilege evidence.
The only way to ensure effectiveness is to invest in building and using reliable evidence. The stakes are high, public dollars are scarce, the public is cynical — and lives and livelihoods hang in the balance.
What Is “Reliable Evidence?”
What does “reliable evidence” mean? After all, the phrase is often bandied about loosely. It means evidence from an independent study using a reliable research design, demonstrating that the program produces a net difference above and beyond what would have happened anyway.
The key idea here is “making a net difference above and beyond what would have happened anyway.” To determine whether a program is making a net difference, one needs to identify a comparison group that shows what would have happened to the same people if the program did not exist.
Why is this necessary? Isn’t it enough to see an improvement over time in test scores, or in graduation rates, or in employment rates? It is not, as these three examples illustrate:
- Maturation bias: Children grow, mature, and develop over time. They get bigger physically, they learn new things, they know more at the end of the school year then they knew at the beginning. Even juvenile offenders age out of crime. The challenge is to avoid giving programs credit for what would have happened anyway through simple aging.
- History bias: Consider an employment and training program where success is measured by the job-retention rate one year later. One year into the program, the job-retention rate has fallen from 50 percent to 40 percent. That looks like a failure, but what if that year spanned from the summer of 2008 to the summer of 2009, in the depths of the Great Recession? Maybe the job-retention rate would have fallen more in the absence of the program. Without a comparison group, one has no way to gauge the effect of the program independent of the effect of the economy.
- Selection bias: This relates to how one selects that comparison group. The first impulse might be to use a group that did not volunteer for the program or whose members were turned down for one reason or another. The problem with this is that volunteers are different from people who do not volunteer. They are more highly motivated, for example. Similarly, people who are screened out of programs because they do not meet certain criteria are by definition different from people who do meet those criteria.
It turns out that random assignment is a simple, elegant, and reliable solution to these three biases. That is why the Food and Drug Administration requires results from a randomized controlled trial before approving a new medicine, and it is why such designs are considered the gold standard of evaluation. But random assignment designs are not always feasible, and evaluators are sometimes forced to settle for second-best designs.
This is not an argument for evaluators only. Philanthropies and government entities also have roles to play in assessing and funding reliable evidence. They can start by asking: Is there a comparison or control group? Does it really control for maturation bias and for history bias? How does the program enroll new clients? Is there a lot of screening at the outset? Is the comparison group different from the program group in some fundamental way (for example, in its motivation)? How might these things affect the reliability of the results?
They can also commit to evidence building as a process. Evidence is not meant to lead to an up-or-down decision. Rather, it should contribute to a continuous process of trial and error and advancement. Done well, it provides a blueprint for program improvement. Often a first evaluation will find that a program is making only a small difference. But the details of that evaluation can address whether the program is addressing underlying causes, whether it is serving the right group of people, whether clients are engaged, whether the staff assembled is right for the program, whether the materials and curriculum are right for the problem, whether there is adequate support in place for program graduates, and much more. The answers to these questions can help the program make a bigger difference in the future.
Using Evidence in Program Expansion
The evidence-building process should also continue as programs are expanded to a large scale (see A Funder’s Guide to Using Evidence of Program Effectiveness in Scale-Up Decisions). There are new challenges associated with taking a small-scale program that is making a difference and expanding it to a large scale. Many models and programs backed by strong evidence have foundered at this stage. Whether or not the program is successful at building large-scale systems or integrating itself into existing large-scale systems will ultimately depend on good information about what difference the program is making at a large scale, and how and why.
This information can be used in two ways. In the first paradigm, evidence contributes at each step of the program’s evolution and expansion. In the second, evidence is used to improve a program model already operating on a large scale.
The Nurse Family Partnership (NFP) provides a good example of the first paradigm. NFP is a nurse home-visiting program aimed at helping the firstborn children of low-income families get off to a healthy start. Its intervention starts during pregnancy and extends into the first year or two of a child’s life. Nurses visit families at home, assess children’s health and development, and offer advice and guidance on breast-feeding, sleep patterns, introducing solid foods, developmentally appropriate activities (like identifying black-and-white patterns and shapes), getting regular checkups, and avoiding secondhand smoke. David Olds, the developer, identified a problem, came up with a concept, pilot-tested a response, and built a model into an operating program over two decades of repeated trial and error, operation, and research. Currently serving nearly 30,000 families, NFP is now being expanded to an even larger scale with support from the Affordable Care Act, which included $1.5 billion over five years for evidence-based home visiting programs. The plan for expansion includes a careful evaluation of the program’s effects at a large scale, an evaluation that was mandated by Congress and that has been designed to inform program improvement.
Reading Partners is following a similar path, although it is earlier in its journey. Reading Partners is a voluntary tutoring program for elementary school children struggling with literacy. Its model has support from an early study conducted by researchers at Stanford that was encouraging but that left open many questions. With support from the True North Fund, the program has expanded to seven states and the District of Columbia, and an evaluation of the expansion has just concluded. The results demonstrate that this low-cost program using old-fashioned tutoring methods is helping children who are behind to catch up with their peers. The final report will also offer lessons for strengthening the program.
Meanwhile, the preschool arena offers a good example of the second paradigm for using evidence to inform large-scale implementation. Over the years, the extraordinary results of early tests like Perry Preschool and Abecedarian have led to large-scale expansion of preschool programs like Head Start that promise to help 3- and 4-year-olds catch up to their more-advantaged peers. Unfortunately, national evaluations have repeatedly found the effects of these large-scale programs to be small and to decay within a year or two. Yet the United States spends $8 billion on Head Start alone. Clearly more needs to be done to make the most of this extraordinary investment. Evidence can contribute in two important ways.
First, evaluations can test new approaches to key parts of the curriculum and pedagogy. For example, they can test new approaches to teaching and modeling social and emotional development for children or new approaches to numeracy as a way to strengthen executive-functioning skills like working memory. Interestingly, early numeracy instruction that builds on the math concepts children know innately (size, shape, distance, and time) actually appears to improve reading as much as literacy instruction. Evaluations could also experiment with structural elements, like a full day of preschool compared with a half day, or two years compared with one year.
Second, researchers can examine more closely the overall modest effect of Head Start to see if it is masking important variations. What if that modest average effect actually reflected the fact that 25 percent of the programs were high performers making major differences in the lives of children while 30 percent were poor performers? If it were possible to explain why and how the high performers differed from the low performers, that would yield a program-improvement agenda that could substantially raise the average effect of Head Start and other preschool programs, changing the lives of many low-income children.
Conclusion
Evidence has a critical role to play. Making the world a better place means doing things that work, and it means maintaining that effectiveness when programs expand to a large scale.
At the federal level, the White House Office of Management and Budget is demanding good evidence relentlessly. It is behind the design of the Department of Education’s Investing in Innovation program, the Social Innovation Fund, the Affordable Care Act’s approach to home visiting, the Department of Labor’s Workforce Innovation Fund, and the design of teen pregnancy prevention programs. Each is structured so that the bulk of the funding goes to those programs with the strongest evidence.
Given the symbiotic relationship philanthropy has with government when it comes to expanding and sustaining evidence-based programs, it is crucial that philanthropic organizations do the same. It takes courage. The process can be painful, as evidence can sometimes reveal that one’s favorite programs are not making the difference one thought. But done right and with an equal commitment to improving programs that do not initially measure up, it can make a real difference — as part of a drive for collective impact, as a component of Social Impact Bonds, or in marshaling the power of a social movement.
As MDRC’s recently released paper, Boosting the Life Chances of Young Men of Color, makes clear, there is much to build on and to expand. The evidence base is growing in other areas as well — from home visiting to preschool, from the early teaching of reading and math to the rising graduation rates of small high schools of choice, from programs that facilitate the transition from high school to higher education to college reforms that dramatically accelerate and increase degree attainment. But this is a race that can only be won by marathoners; not by those who insist on sprinting.