Using a Regression Discontinuity Design for Evaluation Studies
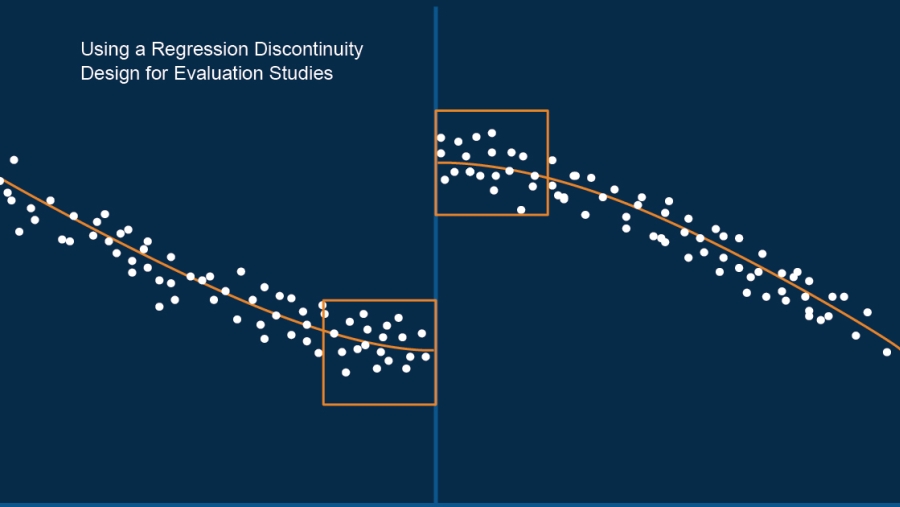
This post is one in a series highlighting MDRC’s methodological work. Contributors discuss the refinement and practical use of research methods being employed across our organization.
A random assignment research design is guaranteed to produce unbiased estimates of program effects, but random assignment is not always feasible. Some individuals, organizations, or communities may view it as unfair or may be reluctant to deny their neediest participants access to an intervention that could prove beneficial. In other cases, random assignment is not possible because the program is already being implemented. Therefore, it is imperative that the evaluation field continue to pursue alternative rigorous designs. One such approach that has seen widespread interest in recent years is regression discontinuity design (RDD).
RDD applies to situations in which candidates are selected for treatment based on whether their score or rating in some area falls above or below a designated threshold or cut point — for example, students chosen for a scholarship based on grade point average. The threshold creates a “discontinuity” in the probability of receiving the treatment. Under certain conditions, comparing people just above and below the cut point can provide information about the treatment effect.
RDD is appealing from a variety of perspectives. Situations that lend themselves to RDD occur frequently, and one can often use existing data to conduct analyses of program impact. In prospective studies, RDD relies on the selection process already being used to determine program participation. When key assumptions are met (more on this below), when implemented and analyzed properly, and when the results are interpreted appropriately and are generalized to the right population, an RDD can be almost as good as a randomized experiment in measuring a treatment effect.
When is it appropriate to use RDD?
Because RDD is a quasi-experimental approach, it must meet a set of conditions to provide unbiased impact estimates and to approach the rigor of a randomized experiment (for example, Hahn, Todd, and van der Klaauw, 2001). First, the rating cannot be caused by or influenced by the treatment: It must be measured before the start of treatment or be a variable that can never change. Second, the cut point must be determined independently of the rating variable, and assignment to treatment must be largely based on the candidate ratings and the cut point. For example, when selecting students for a scholarship, the selection committee cannot look at which students received high scores and set the cut point to ensure that certain students are included in the scholarship pool, nor can they give scholarships to students who did not meet the threshold.
How has MDRC used RDD?
MDRC first used RDD for the Reading First impact study, which sought to measure the effects of a new $1 billion federal funding stream on teachers’ instructional practice and students’ reading comprehension test scores. The study was originally planned as a randomized controlled trial, in which eligible schools from a sample of districts would be randomly selected to receive Reading First funds or become members of a control group. The approach was not feasible, however, in the 38 states that had already begun to allocate their grants. And in the remaining states, randomization was counter to the spirit of the Reading First program, which strongly emphasized serving the schools most in need.
The study team turned to an RDD that capitalized on the systematic process used by a number of school districts to allocate their Reading First funds. After careful fieldwork, it was determined that the study met the internal validity conditions:
-
Eligible schools were rank-ordered for funding based on a quantitative rating, such as an indicator of past student reading performance or poverty.
-
A cut point determined whether schools would receive grants; the cut point was set in each district without knowledge of which schools would receive funding.
-
Funding decisions were based only on the cut point.
The evaluation compared outcomes for two groups of schools with ratings near the cut point to establish the program impact: schools that were selected to receive a Reading First grant and schools from the same districts that did not receive a grant.
More recently, we applied RDD to the evaluation of Response to Intervention (RtI) practices for elementary school reading. A randomized trial was not feasible because RtI practices had been widely adopted by schools around the country, but the way that RtI schools identified students who struggled with reading and assigned them to more intense reading interventions created the opportunity to use RDD. Reading performance was tested at the beginning of a school year, and a set of predetermined rules was then used to place students in tiers. Students whose test scores fell at or below the cut point were considered at risk and assigned to the more intense interventions. These students constituted the study’s treatment group; students who scored above the cut point were the comparison group. The RDD estimated the impact of the more intense reading intervention by assessing the size of the discontinuity or “jump” in student outcome at the cut point.
Things to pay attention to when using RDD
When considering RDD for an evaluation study, there are a few important considerations. First, it is essential to assess the validity of the design, gathering all relevant information on the process for assigning the ratings and the cut point and conducting data analysis to learn whether they are determined independently. (There are also different types of RDDs, such as sharp or fuzzy RDDs and RDD with multiple rating variables, each with its own assumptions.)
Second, it is particularly important to consider the precision of the estimates that can be obtained from the RDD to make sure that the study has adequate power for detecting effects with policy-relevant and meaningful magnitudes. The power to detect effects is considerably lower for an RDD than for a comparable randomized trial.
Third, the researchers need to consider the generalizability of impact estimates based on an RDD to ensure that the findings will match the study’s research questions. Much of the existing literature contends that the estimated impact applies only to the population at or close to the cut point. More recently an expansive view has emerged, arguing that the amount of random error in the rating variable indicates how heterogeneous the population close to the cut point is, and thus how generalizable RDD findings will be (Lee, 2008): The greater the degree of random error, the more broadly generalizable the findings.[1]
Finally, an RDD can provide rigorous estimates of program impacts only if it is analyzed correctly. This largely hinges on whether the analytic approach correctly captures the relationship between the rating variable and the outcome, which may not be a linear relationship. A variety of parametric and nonparametric approaches have been proposed in the literature (Lee, 2008; Jacob, Zhu, Somers, and Bloom, 2012); there are assumptions involved in each approach, and comprehensive validation and robustness checks are important.
[1]An MDRC paper under journal review presents empirical examples: Howard S. Bloom, Andrew Bell, and Kayla Reiman, “Using Data from Randomized Trials to Assess the Likely Generalizability of Educational Treatment-Effect Estimates from Regression Discontinuity Designs.”