Predictive Modeling of K-12 Academic Outcomes
A Primer for Researchers Working with Education Data
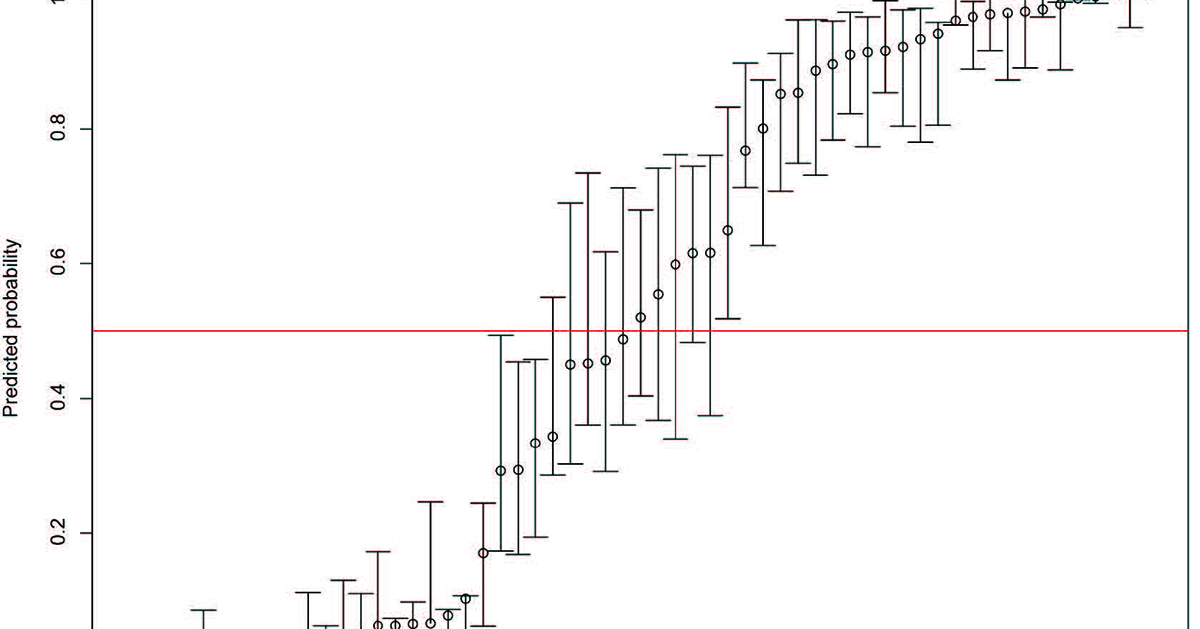
As schools train their attention on identifying students at risk of not meeting key education milestones, researchers are searching for analytic methods to help them do so accurately. One approach is predictive modeling, which estimates individuals’ future outcomes or their risk of not meeting future outcomes. It does this by building and testing a model using data on similar individuals whose outcomes are already known. Commonly used in business and marketing research, predictive modeling is gaining currency in many social policy domains as a way to identify individuals who may benefit from targeted intervention. When results are interpreted correctly, predictive modeling offers benefits to those engaged in continuous improvement efforts and those who are looking to allocate resources more efficiently.
At present, school districts often employ early warning systems, using student-level data, to identify students who are at risk of not meeting key academic outcomes, such as graduating on time or passing state exams. For example, the risk of not graduating from high school is commonly estimated by the so-called ABC indicators of attendance, behavior, and course performance. An indicator-based approach typically produces a binary measure (at risk or not at risk) or a categorical measure of risk (for example, low, medium, or high) based on a snapshot of readily available measures of student behavior and performance. Yet the increasing availability of rich, longitudinal data sets with frequent data updates of many student measures, including daily attendance, exam scores, and course marks, provides an opportunity for district and school staff members to compute more accurate and nuanced predictions of student risk.
MDRC researchers have developed and implemented a comprehensive predictive modeling framework that allows for rapid and iterative estimation of a continuous measure of risk (a probability between 0 and 1 of not achieving an outcome) for each student at a point in time. The framework was developed during a partnership with New Visions for Public Schools (NVPS), a nonprofit organization that works with more than 200 public schools in New York City. The framework was tested with data from a network of 70 high schools to estimate students’ risk of not graduating on time and of not passing the state algebra exam required for graduation.
The primer describes MDRC’s analytic approach.