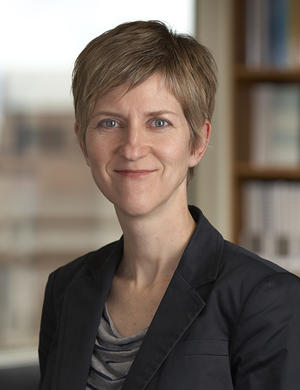
Porter is a statistician with expertise in predictive analytics, machine learning, causal inference, and statistical computing. With over 25 years of experience, she has made significant contributions to quantitative research in K-12 education, work and income security, criminal justice, and public health. As a quantitative methodologist, Porter develops innovative methods and tools for both applied researchers and practitioners working with data. She has served as project director and principal investigator on multiple research methodology grants from the Institute of Education Sciences (IES), focusing on regression discontinuity designs and multiple hypothesis testing. This work has produced open-source software to implement new methods she developed for estimating statistical power when correcting p-values for multiple hypothesis tests. As a data scientist, Porter collaborates with social service providers, leveraging their data to enhance their programs and improve the outcomes of the low-income individuals and families they serve. Porter pioneered the development of MDRC's predictive analytics framework and toolset and continues to lead their ongoing refinement. Currently, she advises integrating predictive analytics into two projects to improve and target services in workforce training programs. With her commitment to advancing applied statistical methods, Porter plays a pivotal role in training and supporting MDRC staff and hosts the MDRC’s Reflections on Methodology blog series. Porter holds a master's degree in public policy from the University of Chicago and a PhD in biostatistics from the University of California, Berkeley.