Pairing Predictive Analytics with Implementation Research
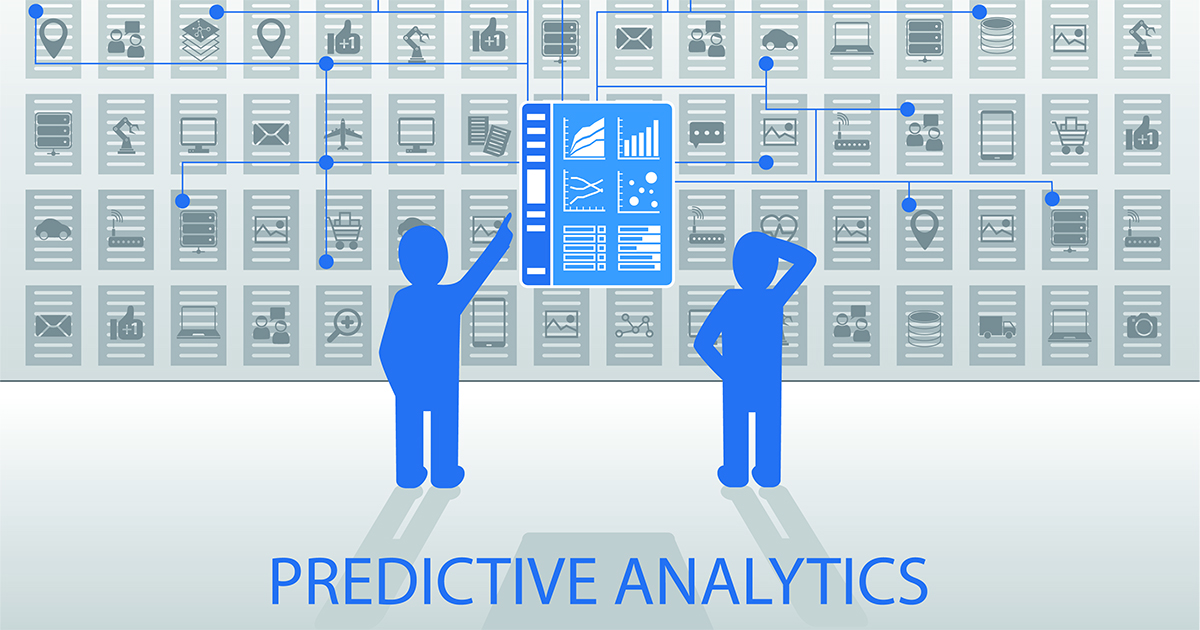
A growing number of public, nonprofit, and for-profit organizations, in an effort to target resources effectively and efficiently, are turning to predictive analytics. The idea of predicting levels of risk to help organizations identify which staff members or clients need support appeals to program managers and evaluators alike. MDRC has been working with organizations to develop, apply, and learn from predictive analytic tools. (Our practitioner brief and primer for researchers provide further background on these methods.) A key insight from our application of predictive analytics is its power to jump-start conversations about program implementation and practice, suggesting that it will become increasingly important for implementation researchers to understand.
The basics of predictive analytics
Many education and service organizations already classify clients in terms of who is at risk of needing intensive services or not completing a program. Predictive analytics moves beyond systems that classify people as either at risk or not at risk. Instead, the approach creates a continuous measure that enables an organization to rank individuals by their risk of adverse outcomes, such as course failure or recidivism. Risk levels can be estimated using a simple logistic regression model with a limited number of measures, or they may involve complex machine-learning models with hundreds of measures. Either way, predictive analytics can identify the types of clients who could most benefit from an intervention or describe patterns of program participation (or absence) that are correlated with risk.
Note that these purposes address who is at risk, not which interventions are effective or for whom particular interventions are most effective. In short, predictive analytics identifies opportunities to intervene and with whom, but not interventions themselves.
Implementation research can inform the use of predictive analytics
The use of predictive analytics in an organization may fundamentally change practice, organizational culture, and the structure of work by reordering the priority of clients and services. Accordingly, a number of implementation research (IR) questions may be relevant depending on the scale and scope of the predictive analytics effort.
Before the adoption of predictive analytics, IR can:
-
Assess institutional readiness to use predictive modeling for decision making (for example, the likelihood of use and views of legitimacy of the process, or how processes related to staff training or client eligibility might change based on predicted risk levels)
-
Identify measures that are important to include in the predictive model (for example, measures that are available in administrative data, or that are viewed as valuable by frontline staff members and leaders), as well as those that are not useful to include in the model (such as measures that are not reliably collected and recorded)
-
Assess to what extent decision making and service provision currently rely on perceptions or data-driven classifications of risk (for example, if a program has a limited number of slots, does the program offer services only to those considered high risk, or is risk considered after a client is accepted?)
-
Assess to what extent predictions of risk will add value beyond the approach currently used
-
Determine whether the organization has a plan to communicate the results of predictive analytics to its staff, by what means (in a dashboard?), and how often
-
Assess the availability of suitable interventions (is there an opportunity for practitioners to act on information about risk?)
During and after the adoption of predictive analytics, IR can:
-
Assess whether and how predictive analytics was incorporated into practice (for example, was it used by administrators, technical staff members, or frontline staff members?), addressing both intended and unintended consequences
-
Document staff experiences with and views of predictive analytics, including whether its use can be sustained
-
Identify structural or process factors that impeded or fostered adoption of predictive analytics (such as dedicated data teams) or impeded or fostered use of the results
-
Assess how organizational culture impeded or fostered use of the results
Use of predictive analytics has the potential to improve program effectiveness; IR can help programs make the most of this potential. And as organizations increasingly use more sophisticated screening or risk assessments in their decision making, implementation researchers can shed light on how the use of these tools shifts practice and culture.
Suggested citation for this post:
Balu, Rekha, and Kristin E. Porter. 2017. “Pairing Predictive Analytics with Implementation Research.” Implementation Research Incubator (blog), September. http://www.mdrc.org/publication/pairing-predictive-analytics-implementation-research.